
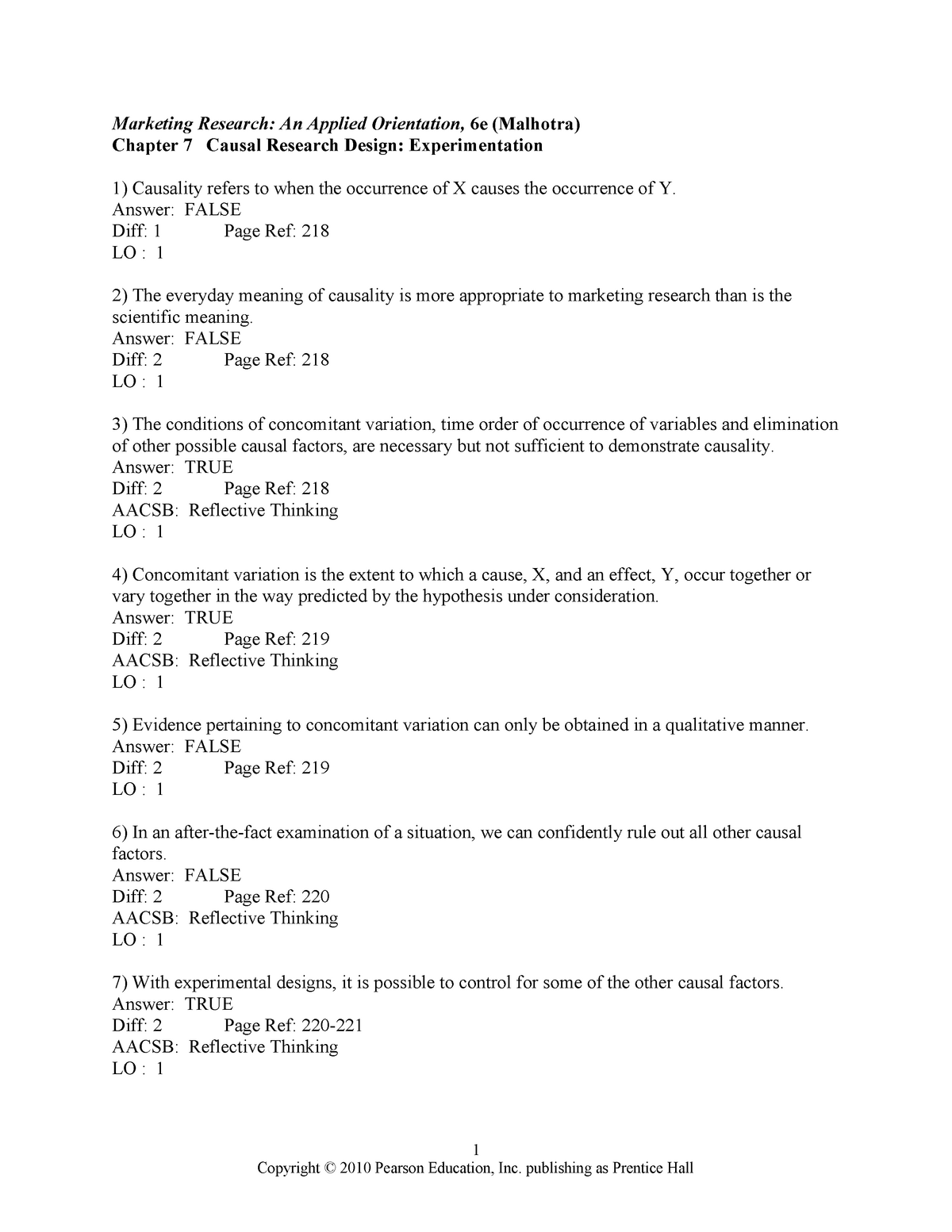
📚 Download the playbook > How to test for causation in your productĬausal relationships don’t happen by accident. Read our playbook for expert advice on tools, strategies, and real-world examples to improve user retention.

RETENTION PLAYBOOK To grow your product, you need a strong retention strategy. All you know is that the two are correlated. The rational you knows that you don’t have enough information to conclude whether joining communities causes better retention. This seems like a massive coup.īut hold on. By Day 7, you see 60% retention in community-joiners and about 18% retention for those who were not. Nearly 90% of those who joined communities are still around on Day 1 compared to 50% of those who didn’t. Your analysis reveals a shocking finding: Users who joined at least one community are being retained at a rate far greater than the average user. One cohort only has users who joined communities, and the other only has users who did not join communities. Curious about whether communities impact retention, you create two equally-sized cohorts with randomly selected users. You ask your team to develop a new feature that allows users to join “communities.”Ī month after you release and announce your new communities feature, adoption sits at about 20% of all users. You make the key bet that user retention for your product is linked to in-app social behaviors. Picture this: you just launched a new version of your mobile app. You might expect to find causality in your product, where specific user actions or behaviors result in a particular outcome. There is a chain reaction: A causes E, which leads E to cause B (but you only saw that A causes B from your own eyes).Īn example of correlation vs.There’s another variable involved: A does cause B-as long as D happens.The two are correlated, but there’s more to it: A and B are correlated, but they’re actually caused by C.
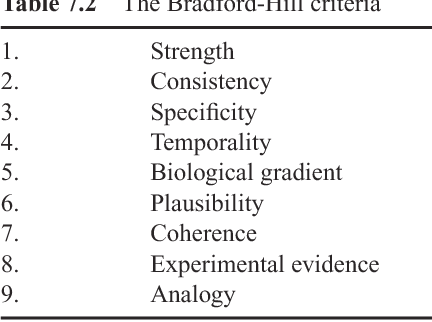
The opposite is true: B actually causes A.Two, there are so many other possibilities for an association, including: One, our observations are purely anecdotal. However, we cannot simply assume causation even if we see two events happening, seemingly together, before our eyes. That would imply a cause and effect relationship where the dependent event is the result of an independent event. We often fabricate these patterns when two variables appear to be so closely associated that one is dependent on the other. Action A relates to Action B-but one event doesn’t necessarily cause the other event to happen.Ĭorrelation and causation are often confused because the human mind likes to find patterns even when they do not exist. On the other hand, correlation is simply a relationship. Causation explicitly applies to cases where action A causes outcome B. While causation and correlation can exist at the same time, correlation does not imply causation. What’s the difference between correlation and causation? Two robust solutions your team can use to test for causation.The key differences between correlation and causation.Know the key differences between correlation and causation.Product managers, data scientists, and analysts will find this useful for leveraging the right insights for product growth, such as whether certain features impact user retention or engagement. In this piece we are going to focus on correlation and causation as it relates specifically to building digital products and understanding user behavior. But recognizing their differences can be the make or break between wasting efforts on low-value features and creating a product that your customers can’t stop raving about. Correlation and causality can seem deceptively similar.
